Businesses are in despair dealing with endless customer expectations. People demand faster and swifter online shopping experiences, today, due to all the advancement in e-commerce. That’s because shoppers, today, expect personalization. Everywhere. Studies show that 47% – 76% of shoppers anticipate personalized interactions with brands. When done effectively, it can increase your revenue by almost 10% or even more. To cope up, many businesses want to use AI-driven personalization to level up their customer experience (CX) strategies.
AI personalization requires appropriate application of small language models (SLM) and large language models (LLM) to enhance personalization and greet customers with relevant responses.
If you’re wondering what SLMs and LLMs are, and how they can help you personalize customer experience for your brand, hang tight. Scroll through to get insights on how these two models come together to create a powerful hybrid.
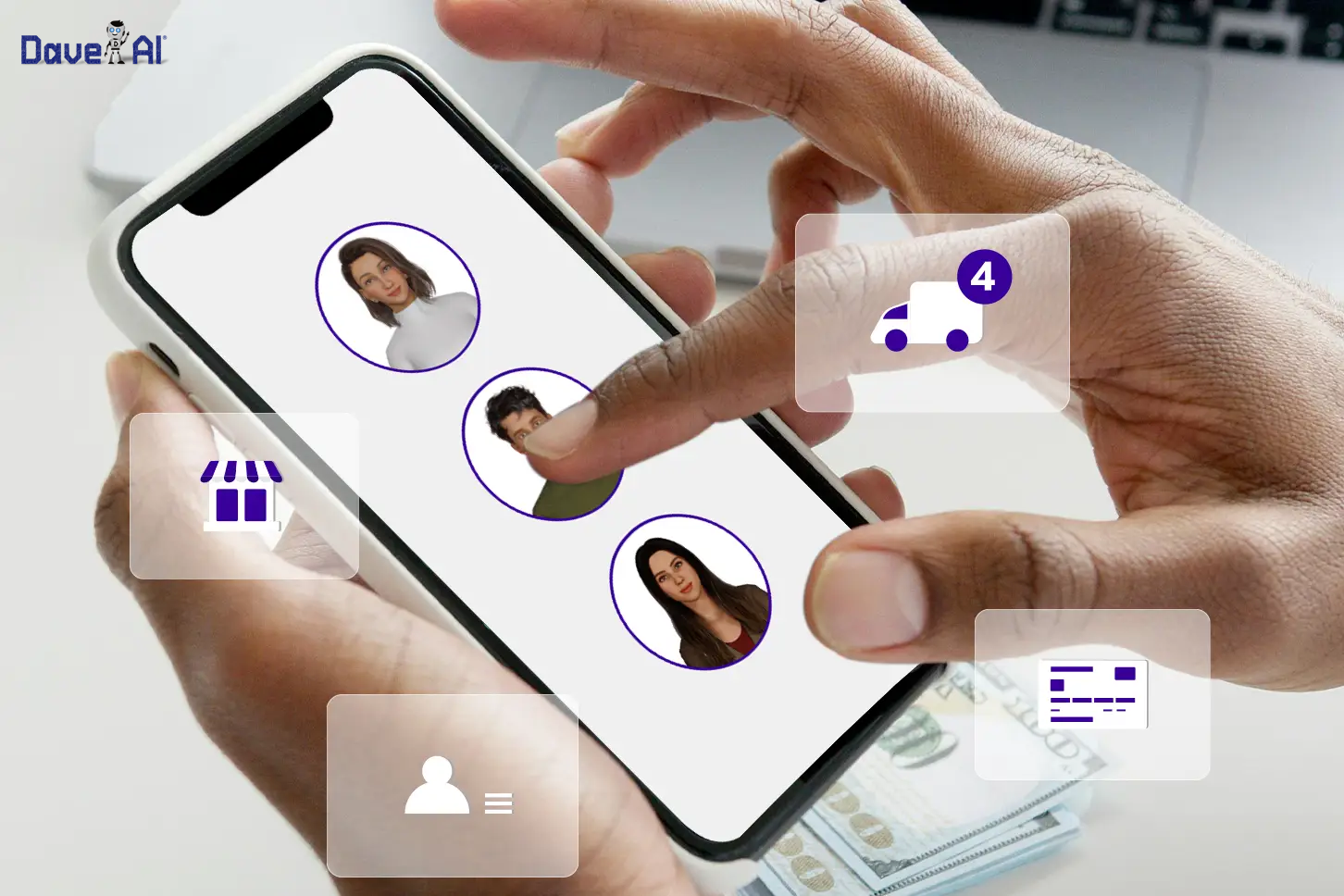
What Are SLM and LLM?
Understanding Small Language Models (SLM)
Small Language Models (SLMs) refer to compact artificial intelligence systems made for natural language processing tasks. Unlike their larger counterparts, SLMs are trained on around 1 billion parameters, which make them more efficient with energy consumption and computational resources. These models have the ability to balance performance with size, often using techniques like pruning, distillation, or productive architecture designs.
SLMs can perform various NLP tasks like text generation, translation, and sentiment analysis, but with potentially reduced capabilities compared to LLMs. Their smaller size enables deployment on edge devices, quicker inference times, and improved accessibility, making them perfect for applications where there are limited resources or privacy is a concern.
As an example, an SLM-based system integrated into an online retail setting, can help store customers find specific products. Is your customer looking for a smartphone? SLM already has phone accessories recommendations ready for them.
Exploring Large Language Models (LLM)
A large language model (LLM) is a powerful AI system trained on billions and trillions of parameters. Think of them as a super-intelligent computer program that has read countless articles, books, and websites, learning relationships and patterns. This allows LLMs to generate human-like text, write different types of creative content, translate languages, and answer your questions in significantly more detail than SLMs.
How do they do that? Large language models have in-built enormous neural networks, mainly in transformer architectures, such as in GPT-4 and BERT. Transformers work well on sequences, and therefore, they are suitable for language applications. They aid LLMs in outperforming deep learning tasks such as text summarization or natural language generation like a pro.
Keep in mind, though, that unlike Small Language Models, LLMs require more computing power and resources to run and manage.
Why Combine SLM and LLM?
When combined, SLMs and LLMs result in a hybrid model that can help organizations perform better at tasks. Small models are excellent for simpler, resource-efficient natural language processing tasks, like keyword analysis or simple data classification. The larger counterpart, on the hand, can prove effective at text generation and answering more complex questions.
Moreover, with a hybrid approach, you can save on costs—SLMs will do the small work here, saving computational power, while LLMs can be deployed in case of more complex queries. This approach also speeds up responses, and enhances privacy since SLMs can handle sensitive data locally while LLMs tackle broader tasks in the cloud.
Key Applications in Customer Experience (CX)
What do SLM & LLM do to enhance CX? Both models have special capabilities that can turn around CX for your brand. Let’s see how.
Efficient Customer Query Resolution
Let’s say a food delivery app has an SLM deployed. This advanced system can check up on questions related to the order status, approximate delivery time, and more. It quickly provides delivery details to customers, and assists with such routine tasks.
This is possible because SLMs specialize in answering repetitive customer queries by spending relatively fewer computational resources.
Complex queries, on the other hand, are seamlessly tackled by LLMs, which can provide detailed, context-aware answers, such as helping customers decide what to order based on their health condition.
Deep Insights with Contextual Understanding
LLMs can fetch detailed insights on customers’ behavior and even sentiments. It analyzes their inputs and understands contexts. For example, a lot of brands want to conduct product/service surveys. LLMs can create and analyze such customer surveys based on their command of natural language.
Plus, they can perform sentiment analysis. It lets you gauge online shoppers’ pulse across various sources, like social media, customer feedback and reviews. This kind of detailed information tells you how happy or satisfied your customers are.
Multilingual Customer Support
Thirdly, if you have customers from around the world, who speak different languages, you’ll likely need multilingual support.
SLMs can manage basic tasks like detecting the customer’s language, routing queries to appropriate resources, or handling common phrases in multiple languages (e.g., “Where is my order?”).
LLMs, with their extensive training on multilingual datasets, can handle complex interactions in almost any language. They excel at generating contextually relevant and grammatically correct responses, even in less commonly spoken languages or when switching between languages mid-conversation (code-switching).
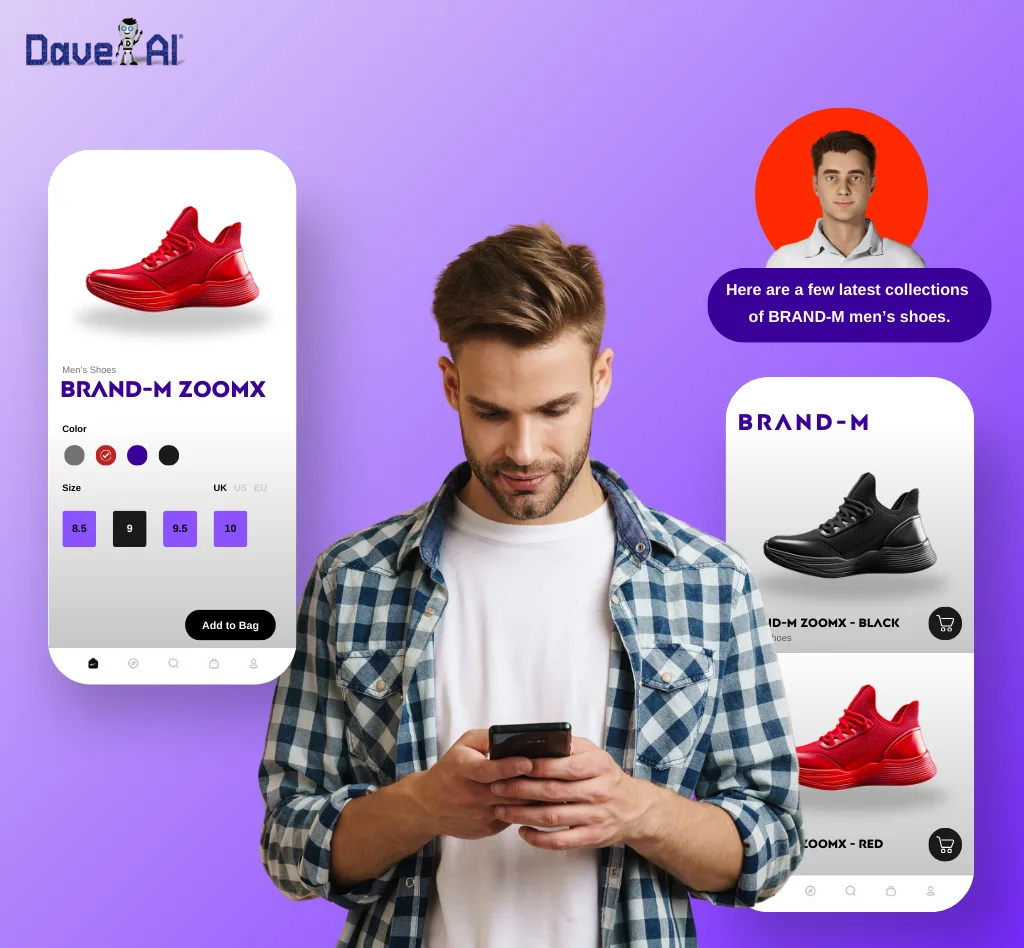
Transforming Personalization with SLM + LLM
Your customer just bought a jacket from your online store, now you want them to see similar or related things when they visit your e-shop again. It’s a valid demand, but involves studying your buyer’s behaviour.
That’s where the two personalization heroes (SLM & LLM) jump in to transform it for your brand through artificial intelligence.
Personalized Recommendations
You may have stumbled upon items being suggested to you during online shopping. These are most likely products that you’d be inclined to buy considering your purchase history. SLMs
can provide such targeted recommendations with their ability to quickly scan specific data points, including buyer’s preference, search history, or purchasing patterns, and make relevant suggestions.
Meanwhile, LLMs help customize these suggestions according to different, individual customer profiles as a medium for adding personal touch.
Say, a customer recently bought running shoes. The SLM will process this information quickly and make suggestions for related products, like a water bottle or workout gear, based on the purchase history of this buyer. The model identifies these patterns and associations in smaller, specific datasets to provide such recommendations swiftly.
The LLM, in this example, would consider broader contexts, like the customer’s fitness goals or seasonal trends, to enhance the personalization experience. For example, it can recommend lightweight apparel for outdoor activities, if it’s summer time. SLM and LLM here assess the buyer’s current and future needs, adding the most valued personalized touch to CX.
Advanced Behavioral Predictions
Another feature of a hybrid model involves analyzing customer behavior and making predictions accordingly.
Large models gauge historical customer data to predict behavior patterns. SLMs then use these predictions for accuracy and relevance in real-time interactions.
Think about how Netflix recommends a movie just when you’re bored. It’s AI-driven personalization working behind-the-scenes.
Cross-Channel Consistency
You need a lot of consistency across different marketing channels, including email, apps, and websites for effective personalization. You’ll be able to achieve it effortlessly when small language models handle platform-specific interactions, like chatbot responses, and LLMs manage overarching customer insights.
Industry-Specific Applications of SLM + LLM
SLM & LLM together transform various industries in different ways. Let’s check out how.
Retail and E-Commerce
Virtual shopping assistance, anyone? Most buyers, these days, are looking for, or rather expect it in an online store. SLMs do the job well by helping customers find products in an instant. Meanwhile, LLMs analyze buying patterns and preferences and support upselling.
Banking and Financial Services (BFSI)
Customers need practical guidance to make profitable investments or financial decisions. In a hybrid model, LLMs can give them in-depth financial consultations, like retirement planning or investment advice, to help out. Alternatively, SLMs can provide quick guidance on secure transactions or account balances. It boosts customers’ confidence and also saves their time.
Healthcare
AI personalization in healthcare is a total game changer. Patients feel more cared for and the system gains increased efficiency levels. With a hybrid system, companies can use SLMs for the most mundane patient FAQs and tasks, like scheduling appointments. At the same time, LLMs help fetch diagnosis suggestions based on the patient’s medical history.
Hospitality and Travel
With a hybrid approach in this industry, SLMs can help address simple booking inquiries, like room availability or flight schedules. LLMs can take on a more detailed, creative task of curating personalized travel itineraries according to customer preferences. It only leaves happier customers behind with customized vacation solutions.
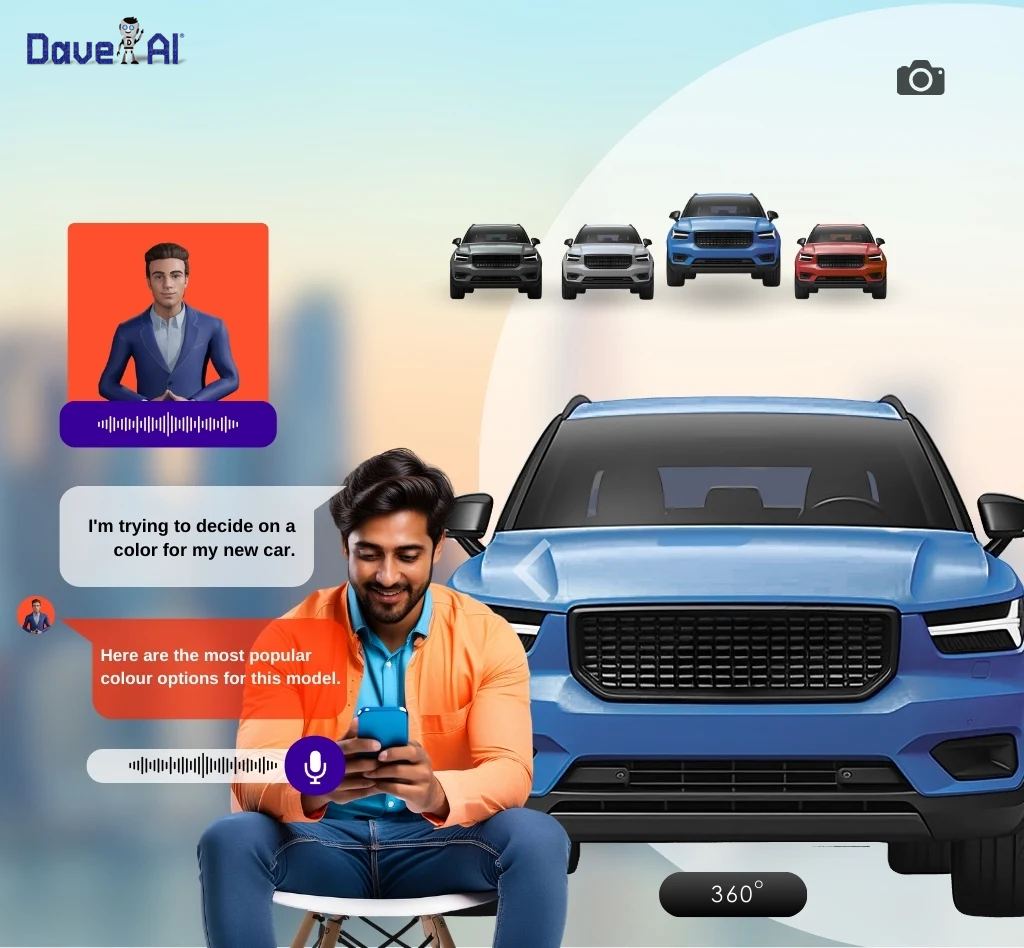
Benefits of Using SLM + LLM in CX
Below mentioned are a few advantages of using a hybrid system:
Speed and Efficiency
You can expect rapid query resolution, especially for repetitive enquiries with SLMs. Along with reducing customers’ wait times, it also improves their satisfaction levels. Plus, using AI-powered knowledge bases can considerably cut customer support ticket volumes as customers are able to find answers independently of support teams. This means fewer frustrated customers waiting for answers and more time for your team to handle complex queries.
Scalability and Depth
LLMs, as part of the hybrid model, help companies deal with complex customer needs. They quickly comprehend broader contexts to do so. It makes them perfect for industries that need intense problem-solving and personalization. Market Research says an e-commerce platform might see more than 30% sales uplift when recommendations are tailored to individual preferences. Customers feel seen, and your business thrives—it’s a win-win!
Cost Optimization
The hybrid model also brings some relief to your entrepreneurial pockets by saving costs. For example, an IBM report says that using chatbots can downsize customer service costs by up to 30%.
As you automated the routine tasks, it helps cut down on operational expenses. Hybrid AI systems manage enormous volumes of data and tasks at a fraction of the time and cost a human would usually take to accomplish it. This way it allows businesses to reallocate resources strategically and invest more time and money in growth-prone areas.
Challenges and Solutions
Although you may see many benefits coming in the package with a hybrid model, but you may face some difficulties in implementing it:
Managing Computational Resources
The biggest challenge with LLMs is that they are quite resource-intensive. It can be very costly to train and maintain LLMs. Training them requires massive datasets, extensive computational power, and substantial energy resources. Even deploying LLMs for real-time applications can strain infrastructure and the enterprise’s computational resources.
But, if you go for a hybrid approach where SLMs handle lightweight tasks and LLMs tackle complex queries, it will reduce the computational burden on the system. This needs a proper balance in resource allocation, with smaller models managing repetitive or simpler tasks and larger ones only stepping in when nuanced context or deeper insights are required.
Data Privacy Concerns
With personalization come privacy concerns as these two don’t completely mingle and it’s easy to cross that boundary. Companies may face security concerns, especially with LLMs, like the risk of using and breaching sensitive or confidential customer data.
A business’s duty is to tread carefully and use customer data responsibly by taking explicit consent from customers on data collection and use. You need stringent data security measures to keep things ethical and also promote transparency in how you’re going to use this data.
Thus, both SLMs and LLMs must be trained on anonymized data to protect customer information and ensure an ethical AI deployment.
Seamless Integration
It can be a daunting task to Integrate SLM and LLM into existing systems. There can be many issues during the integration, like:
- Existing systems may not be designed to handle SLM’s and LLM’s dual functionality.
- You require customized configurations to align the capabilities of SLM and LLM, which can be resource-intensive for your enterprise.
- Moreover, there’s often a need to restructure datasets or create new pipelines to feed the models. It can be pretty labour-intensive to have SLMs and LLMs process and interpret the same data formats without loss of information.
But, tools like hybrid AI architectures align models with business needs to simplify this process.
How DaveAI Leverages SLM + LLM for Personalization
DaveAI is an AI platform to help your business create immersive and personalized customer experiences powered by AI sales avatars, chatbots, and more. If you’re searching for tools to make your CX memorable and alluring, you can harness the potential of DaveAI’s tech architecture to seamlessly integrate advanced AI solutions into your existing systems.
Here we’re spilling the beans about how we leverage SLM & LLM for personalization:
Context-Aware Conversations
DaveAI uses SLMs for quick, no-fuss answers and LLMs for deeper, more engaging conversations through AI chatbots. Be it a simple query or a detailed discussion, customers get accurate and precisely trimmed information in no time.
Adaptive Learning for Customer Preferences
Here’s where it gets even smarter—DaveAI solutions constantly learns from real-time feedback. Both SLMs and LLMs adapt and improve. It turns every interaction into a more personalized one, DaveAI Enabled Experiences have which is relevant over time. 30% more lead qualification rates & Dave Qualified Leads have 18% better conversion.
Industry-Specific CX Solutions
Be it any industry; automotive, BFSI, retail, or real estate—DaveAI has AI-driven solutions to tackle specific challenges and uncover unique opportunities in each one. You get perfectly tailored, personalized experiences for your customers.
For instance, If you want to ace customer interactions, DaveAI is a conversational AI platform with Automated Speech Recognition, Natural Language Processing and Speech to Text generation capabilities.
Need product visualizations for your retail e-store? We present you with a state-of-the-art 3D Visualization capability to curate compelling product visualizations and high quality visualizations in low GPU devices.
Want to give that personal touch in CX? We give you an online learning genetic algorithm that is a multi-dimensional affinity engine which predicts affinities between customer & product attributes.
Check out all of DaveAI solutions here.
The Future of SLM + LLM in Customer Experience
We can expect much more sophisticated personalization strategies in different industries as AI and machine learning evolve. These technologies are expected to enable even more profound understanding of consumer behaviors and preferences. It will allow predictive personalization that anticipates customer needs before they even articulate them.
Furthermore, AI and machine learning enhancements will make real-time, adaptive customization of shopping interfaces possible. It’ll also help with predictive stocking and better logistics planning.
Innovations like nuanced sentiment analysis, dynamic pricing models fitting individual purchasing power and habits, and real-time customization of user interfaces further fade the lines between individual consumer desires and personalized online experiences.
If you’re all in for revolutionizing your CX strategy, DaveAI can help you harness the full potential of AI-driven personalization with chatbots, virtual avatars, virtual stores, and more. Reach out to us and book a demo today!
SLMs are lightweight models made for specific tasks, while LLMs are more versatile models that can handle diverse and complex interactions.
SLM provides speed and precision, and LLM adds depth and scalability to the stack to enhance CX.
Combining the two forms a hybrid that provides more efficiency, scalability, and resource optimization for a superior CX.
If you’re worried about security, rest assured that when trained with proper safeguards, both SLMs and LLMs keep data safe.
Sectors benefiting from these two models include Retail & e-commerce, BFSI, healthcare, hospitality, real estate and travel, leveraging SLM & LLM for CX betterment.
DaveAI is a full-stack AI platform to help you create immersive and personalized CX powered by AI sales avatars. WeI transform CX through context-aware conversations, adaptive learning, and industry-specific solutions.
Shashank Mishra
Shashank is an experienced B2B SaaS writer for eCommerce, AI, productivity, and FinTech tools. His interests include reading, music, poetry, and traveling.