RAG(Retrieval Augmented Generation) is an innovative approach in the field of Generative AI that combines elements of retrieval-based models with generative models. In RAG, the model integrates information retrieval mechanisms to enhance the generative capabilities, resulting in more contextually relevant and coherent outputs. The core idea behind RAG in Generative AI is to leverage pre-existing knowledge stored in a retrievable format, such as a large database of documents or passages. When generating responses or content, the model can retrieve and incorporate relevant information from this external knowledge source. This retrieval mechanism helps the generative model to produce outputs that are not only creative but also grounded in factual or contextually appropriate information.
Tune in to our first podcast episode on Generative AI: Spotlight on the world of Generative AI
RAG models find applications in various natural language processing tasks, including question-answering systems, chatbots, and content creation, where a balance between creativity and accuracy is desired. Advantages of RAG in Generative AI are immense. The integration of retrieval mechanisms in generative models represents a promising direction in advancing the capabilities of AI systems for more effective and context-aware content generation. Approximately 76% of companies that have incorporated generative AI into their operations have noted enhancements in the quality of customer experience.
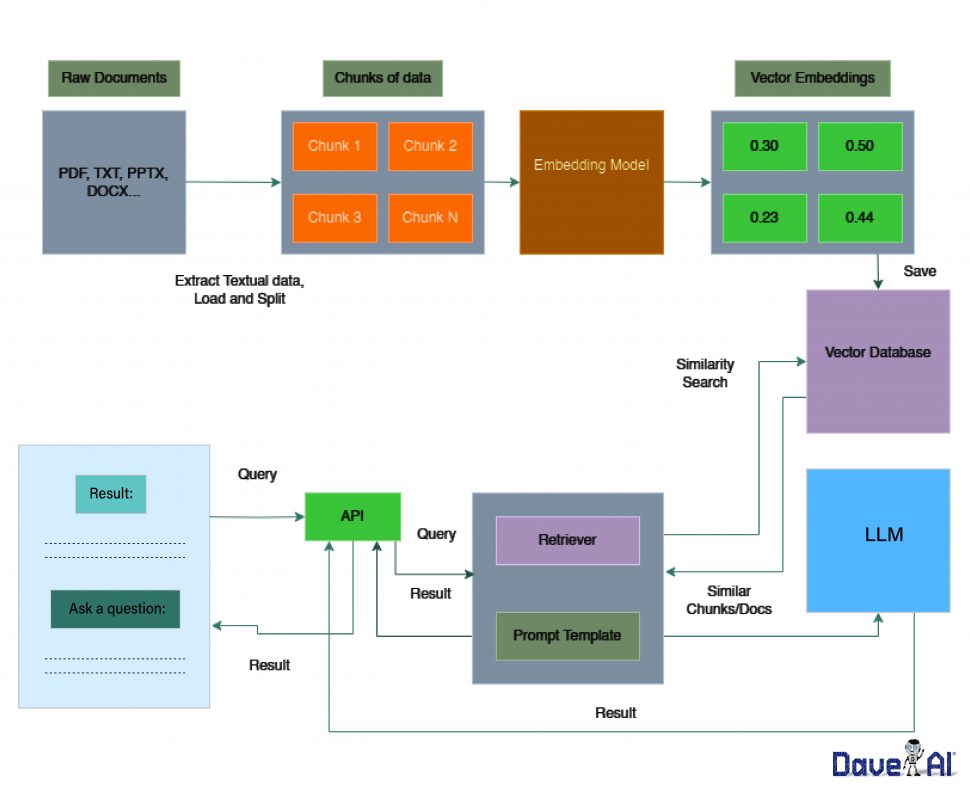
Importance of RAG in Generative AI
RAG stands at the forefront of advancing generative artificial intelligence, offering a transformative approach that combines the strengths of generative models with the richness of external knowledge. The importance of RAG in Generative AI can be elucidated through several key aspects:
- Contextual Relevance: RAG addresses a crucial challenge in generative AI by enhancing the contextual relevance of generated content. The integration of retrieval mechanisms ensures that the model can access and incorporate external information.
- Factually Accurate Outputs: By leveraging a pre-existing knowledge base through retrieval, RAG models contribute to the generation of factually accurate content. This is particularly valuable in applications such as question-answering systems, where access to accurate information is paramount.
- Knowledge Enrichment: RAG enriches generative models with a vast reservoir of external knowledge. This integration enables AI systems to tap into a broader understanding of diverse topics.
- Reduced Bias and Misinformation: Incorporating retrieval mechanisms in generative AI helps mitigate bias and reduce the risk of misinformation. By cross-referencing information with external sources, RAG models can produce outputs that align more closely with accurate and unbiased perspectives.
- Versatility in Application: RAG’s versatility makes it suitable for a wide range of applications, including content generation, dialogue systems, and language understanding tasks. This adaptability positions RAG as a valuable tool across various domains, enhancing the capabilities of generative AI in diverse contexts.
- Closing the Gap with Human-like Understanding: The integration of external knowledge through RAG brings generative AI systems closer to human-like understanding and reasoning. This advancement is crucial for applications where detailed comprehension and contextual awareness are essential.
Role of Language Models in Generative AI
Generative AI language models contribute significantly to the creation of human-like text and facilitate a broad spectrum of applications. Here are key aspects of their role:
- Text Generation: Language models form the core of text generation tasks within Generative AI. They are designed to understand the syntactic and semantic structures of human language, enabling them to generate coherent and contextually relevant text.
- Understanding Context: Generative AI language models excel in capturing contextual dependencies within a given piece of text. This contextual awareness is crucial for generating responses or content that align with the preceding context, providing a more natural and human-like output.
- Diverse Applications: Generative language models, such as GPT (Generative Pre-trained Transformer) by OpenAI, have become instrumental in various applications. From content creation and creative writing to chatbots and virtual assistants, language models power a diverse array of generative tasks.
- Conditional Generation: Generative AI language models can be conditioned on specific inputs or prompts to generate outputs tailored to a particular context or theme. This capability is leveraged in applications like customizing responses in dialogue systems or generating content based on user-specified criteria.
- Multimodal Capabilities: Advanced language models are evolving to incorporate multimodal capabilities, integrating information from various modalities such as text, images, and audio. This enhances their ability to generate content that spans multiple domains and types of data.
- Fine-tuning and Specialization: Generative AI language models can be fine-tuned on specific tasks or domains, allowing them to specialize in generating content relevant to particular industries or subject areas. This adaptability contributes to their versatility in diverse applications.
- Transfer Learning: Pre-trained language models serve as a foundation for transfer learning in Generative AI. Models pre-trained on large datasets can be fine-tuned on smaller, domain-specific datasets to adapt their generative capabilities to specific contexts.
- Creative Writing and Content Generation: Generative AI language models are harnessed for creative writing, content creation, and storytelling. They can generate articles, poetry, scripts, code and other forms of written content with varying tones and styles.
- Conversational AI: In the domain of conversational AI, language models enable chatbots and virtual assistants to understand user queries and generate appropriate responses. This contributes to the development of more natural and engaging conversational agents.
What are the top 5 Multimodal AI models for businesses in 2024? Click to know
Limitations of Traditional Language Models
Traditional language models, especially before the advent of advanced architectures like transformer-based models, had several limitations that impacted their ability to understand and generate human-like text. Here are some key limitations:
- Limited Context Understanding: Traditional models struggled to capture long-range dependencies and complex context in text. They often faced challenges in maintaining a coherent understanding of context over extended passages, leading to issues in generating contextually relevant responses.
- Fixed Vocabulary: Many traditional language models were constrained by a fixed vocabulary. This limitation made it challenging for models to handle out-of-vocabulary words or adapt to evolving language usage and slang.
- Lack of Contextual Awareness: These models lacked the ability to understand the contextual nuances of language. This resulted in responses that were contextually inaccurate or failed to capture the subtleties of human communication.
- Difficulty in Handling Ambiguity: Traditional language models struggled with handling ambiguous language constructs and multiple possible interpretations of a given input. This limitation affected their ability to generate accurate and contextually appropriate responses.
- Inability to Learn Hierarchical Representations: Capturing hierarchical relationships and complex syntactic structures was challenging for traditional models. This limitation hindered their capacity to represent the hierarchical nature of language.
- Fixed Input Length: Many traditional models had fixed input length constraints, making it difficult to process long documents or extensive context. This limitation restricted their effectiveness in tasks requiring a comprehensive understanding of lengthy texts.
- Limited Transfer Learning Capabilities: Traditional language models had limited transfer learning capabilities. Pre-training on one task and fine-tuning on another was often less effective compared to more modern architectures like transformers.
- Difficulty with Multimodal Data: Integrating information from multiple modalities (such as text and images) posed challenges for traditional language models. They were not well-equipped to handle the rich and diverse data types prevalent in modern applications.
- Prone to Biases and Stereotypes: Traditional language models tended to reflect and propagate biases present in their training data. This limitation resulted in models producing biased or stereotypical outputs, contributing to concerns about fairness and ethical use.
- Heavy Reliance on Handcrafted Features: Traditional models often relied on handcrafted linguistic features, which required substantial domain expertise and manual effort. This approach limited the adaptability and scalability of these models to various tasks and domains.
How RAG Differs from Traditional Language Models

- Knowledge Integration: RAG integrates information from external knowledge bases during the generation process, allowing the model to access and incorporate factual information.
- Contextual Enrichment: RAG aims to enrich the context of generated content by retrieving information from a knowledge base, enhancing the contextual relevance of responses.
- Handling Ambiguity: RAG addresses ambiguity by cross-referencing generated content with information retrieved from a knowledge base, providing more precise and contextually informed responses.
- Factually Accurate Content: RAG contributes to the generation of factually accurate content by leveraging retrieval mechanisms to access information from knowledge bases.
- Customization Based on Input: RAG can be customized based on specific inputs or prompts, allowing for the generation of contextually relevant outputs tailored to a particular theme or topic.
- Mitigating Bias: RAG helps mitigate bias by incorporating information from diverse sources during the retrieval process, providing a broader perspective.
- Handling Out-of-Vocabulary Terms: RAG can handle out-of-vocabulary terms better by leveraging external knowledge bases, allowing for more adaptability to evolving language usage.
- Versatility: RAG enhances the versatility of generative models by allowing them to leverage external knowledge for various tasks, such as content creation, information retrieval, and natural language understanding.
- Transfer Learning: RAG demonstrates effective transfer learning capabilities by leveraging pre-existing knowledge bases for specific domains or tasks.
From the desk of our CEO, Sriram PH- CEO Speaks: Navigating The Landscape Of Generative AI
The Components of RAG in Generative AI
A. Retrieval Component: Explaining the Role of Retrieval in RAG:
The retrieval component in RAG plays a crucial role in enhancing the generative capabilities of the model. Its primary functions include:
- Accessing External Knowledge: The retrieval component is responsible for accessing information from external knowledge bases or sources. It retrieves relevant data that can enrich the content generation process.
- Fact Verification: It verifies facts and information retrieved from external sources to ensure accuracy. This step contributes to the generation of factually sound and reliable content.
- Contextual Reference: The retrieval component provides contextual references for the generative process. It helps the model understand the context of the user’s query and retrieve information that aligns with the context.
- Handling Ambiguity: Addressing ambiguity in user queries, the retrieval component cross-references potential interpretations, ensuring that the generated content is contextually informed and precise.
- Diversity in Information Sources: The retrieval component sources information from diverse external knowledge bases, reducing bias and contributing to a more comprehensive understanding of topics.
B. Generation Component: Understanding the Generation Process in RAG:
The generation component is responsible for producing content based on the information retrieved. Its key functions include:
- Contextualized Content Generation: The generation component uses the retrieved information to produce contextually relevant content. It leverages the contextual references to generate responses that align with the user’s query.
- Adaptability and Customization: This component adapts the generative process based on specific inputs or prompts, allowing for customized outputs. It tailors the generated content to specific themes, topics, or user requirements.
- Synergy with Retrieval Information: The generation component works in synergy with the retrieved information to create a seamless flow of content. It ensures that the generated output aligns with the factual details and context provided by the retrieval component.
- Natural Language Fluency: The generation component focuses on maintaining natural language fluency. It ensures that the generated content is coherent, contextually appropriate, and adheres to linguistic patterns.
- Handling Dynamic Inputs: The generation component can handle dynamic inputs, adapting its output to changing information retrieved in real-time. This adaptability contributes to the model’s responsiveness.
C. Fusion of Retrieval and Generation: How RAG Seamlessly Combines Retrieval and Generation:
The fusion of retrieval and generation in RAG involves the seamless integration of the two components to produce high-quality, contextually relevant content:
- Dynamic Information Flow: The retrieval and generation components work in tandem, ensuring a dynamic flow of information. The retrieved knowledge dynamically informs the content generation process, creating a continuous and adaptive loop.
- Contextual Coherence: The fusion ensures that the generated content maintains contextual coherence with the information retrieved. This results in responses that are not only factually accurate but also contextually aligned.
- Real-time Adaptation: RAG adapts in real-time based on changes in retrieved information. This real-time adaptation allows the model to provide up-to-date and relevant content.
- User-Centric Customization: The integration of retrieval and generation components allows for user-centric customization. The model can generate content tailored to specific user inputs, preferences, or themes.
- Enhanced Versatility: The seamless fusion enhances the versatility of RAG across different domains and tasks. It allows the model to leverage external knowledge dynamically, contributing to its adaptability and effectiveness.
Use Cases of RAG in Generative AI
- Content Creation: RAG is valuable in content creation applications, assisting writers and creators in producing contextually rich and factually accurate content. It can be used to generate articles, blog posts, and other written materials.
- Chatbots and Virtual Assistants: RAG’s natural language generation capabilities make it suitable for chatbots and virtual assistants. It enables these systems to understand user queries, retrieve relevant information, and generate coherent and contextually informed responses.
- Educational Platforms: In educational platforms, RAG can assist in generating educational content with accurate and up-to-date information. It supports a dynamic learning environment by adapting to changes in knowledge and providing relevant contextual references.
- Legal and Compliance: In legal and compliance applications, RAG can assist in generating accurate and contextually relevant documentation. It ensures that legal documents are aligned with the latest regulations and specific client requirements.
- Healthcare Information Systems: RAG can be applied in healthcare information systems to generate patient information, responses to medical queries, and educational content. Retrieval from medical databases ensures that the generated content is medically accurate and up-to-date.
- Financial Services: In the financial sector, RAG can assist in generating reports, financial articles, and responses to customer queries. Retrieval from financial databases ensures that the generated content reflects accurate financial information.
- Marketing and Advertising: RAG can be utilized to create marketing content, generate ad copies, and provide information about products or services. By accessing external knowledge sources, it ensures that marketing messages are informed and persuasive.
- Human Resources: RAG can aid in human resources by generating employee handbooks, training materials, and responses to HR-related queries. Retrieval from HR databases ensures that the content adheres to company policies and regulations.
- News and Media Industry: RAG can contribute to news article generation by providing background information, context, and additional details. This ensures that news content is enriched with relevant facts and details.
- Travel and Hospitality: In the travel industry, RAG can assist in generating travel guides, recommendations, and responses to customer inquiries. Retrieval from travel databases ensures that the generated content includes accurate and up-to-date information about destinations.
- E-commerce: RAG can improve product recommendations, product descriptions, and customer interactions in e-commerce platforms. The retrieval component can gather information about products, reviews, and trends, while the generation component can craft personalized and contextually relevant content for users. This application enhances the overall user experience and supports informed decision-making.
Generative AI technologies have seen substantial adoption in the healthcare and finance sectors, where over 47% of companies are actively engaging in the implementation or testing phases of generative AI solutions.
How DaveAI provides an LLM agnostic approach to enterprises?
Generative AI is the most talked about technology today. In just a couple of months it has disrupted various industries. Large Language Models have been the most popular implementation used to improve workflows, assist existing stakeholders and in numerous cases allow customers to converse with an enterprise according to their needs. GPT, for instance, releases faster than ever and up to date models every few months along with a plethora of competitors in the space such as Bard, LLaMA, LangChain. Every enterprise is scrambling to be the first to bring the experience powered by the best LLMs for their customers.
The problem they face is that every time a new LLM is launched or a new version is released, they have to re-engineer their solution to fit the requirements.
There are usually two ways in which LLMs are deployed for enterprise solutions-
a. Prompt engineering occasionally used with RAG(Retrieval Augmented Generation)
b. Fine tuning existing models
Adapting to a new language model incurs a recurring expense in terms of time and effort, as data preparation for either fine-tuning or optimizing prompt-based usage is required. With the GRYD APIs, we provide an LLM agnostic approach to allow users to upload data in form of documents, rest APIs along with rules that the enterprise wishes the LLM to adhere to. Beyond this, the enterprise can use any of our chatbots, 3d avatars, or Rest APIs and switch between LLMs to be used on the fly by changing the model parameter in the query. This means that in the event of a language model failure, performance issues like accuracy or response time concerns, or the introduction of a new model, the modification required is adjusting a single parameter. This removes the need for any re-engineering or fine tuning. This saves time, money and effort with no downside.
https://www.youtube.com/embed/FjuN8aKd0Uo?feature=oembedFor more information, visit- GRYD